Real time flow analysis using machine learning is transforming urban mobilities. Thanks to advances in machine learning, massive volumes of data can now be processed in real-time. This capability enables smarter and more anticipatory management of information.
What is machine learning ?
Machine learning, also known as automatic learning, is a branch of AI that enables systems to process data and learn from it. This technology identifies patterns, predicts behaviors, and makes informed decisions without direct human intervention. Combined with automatic counting technologies, it becomes a powerful tool for detailed, real-time flow analysis.
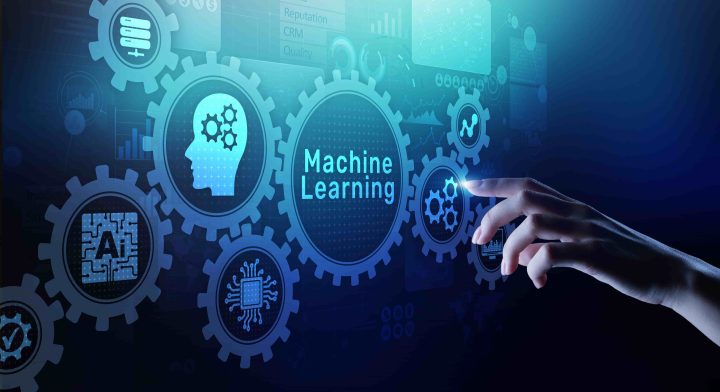
The importance of flow analysis
In urban mobilities, analyzing passenger flows is critical as operators face dual challenges. On one hand, they must optimize resources such as vehicles, infrastructure, and staff. On the other, they are responsible for providing a seamless and pleasant experience for users. This must be achieved amidst the growing complexity of travel patterns, increasing passenger density, and fluctuating demands based on time or location.
Machine learning addresses these challenges by analyzing real time data, providing detailed insights into passenger flows, and highlighting trends and congestion patterns.
Collecting data for machine learning
Machine learning relies on accurate and reliable data, which is where automatic counting technologies come in. These tools capture detailed information on passenger flows, essential for feeding and optimizing algorithms. Smart cameras, widely used in urban mobility systems, analyze passenger flows in real time. They detect and count entries, exits, and movements, even under challenging conditions like high crowds or low light.
Raw data is then processed by machine learning algorithms, transforming it into actionable insights that improve flow management.
How machine learning revolutionizes flow analysis
🔎 Predicting flows to avoid congestion
Machine learning predicts flow variations based on historical and real-time data. Advanced algorithms, such as recurrent neural networks (RNN) or augmented ARIMA models, forecast peak times and adjust schedules or vehicle frequencies accordingly.
💡 Smart resource allocation
Flow analysis powered by machine learning enables efficient redeployment of resources to areas where they are most needed.
🚨 Detecting and responding to anomalies
Passenger flows don’t always follow predictable patterns. Machine learning identifies anomalies like sudden surges, incidents, or unusual behaviors, enabling rapid responses to minimize disruptions.
✔️ Enhancing user experience
Effective flow analysis supported by machine learning offers a personalized experience for users. Real-time information on occupancy rates or alternative routes increases passenger satisfaction and convenience.
Machine learning and automatic counting solutions
Machine learning and automatic counting technologies form a seamlessly integrated ecosystem that delivers dynamic and reliable flow analysis:
- Transforming raw data : Sensors provide rich data, and machine learning algorithms extract clear patterns.
- Increased accuracy : Errors are minimized through cross-analysis of data.
- Real time actions : Managers can adapt resources immediately based on real-time insights.
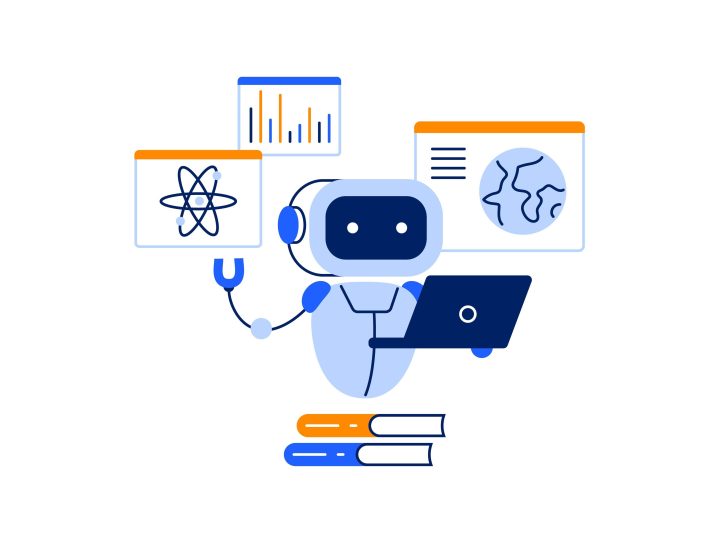
Technical and ethical challenges
Even though it holds great promise, flow analysis using Machine Learning must overcome several challenges. First and foremost, it must protect personal data. Ensuring that the information collected respects passengers’ privacy is essential, in compliance with current laws and regulations, such as the GDPR in Europe. Secondly, it must ensure compatibility between systems. For Machine Learning to perform effectively, it is crucial to integrate various technologies, such as platforms, sensors, and tools, which often come from diverse sources. Achieving seamless and efficient collaboration between these components is a significant challenge for this technology. Finally, AI-based solutions require substantial resources, both financial and human, to implement and maintain.
A promising future for sustainable urban mobilities
Despite these challenges, machine learning offers a unique opportunity to revolutionize flow analysis in urban mobilities. By streamlining travel and making networks more efficient, these technologies contribute to the development of smart and sustainable cities.
Real-time flow analysis powered by machine learning is transforming urban mobilities. This technological combination addresses current challenges while laying the foundation for predictive, responsive, and sustainable mobility.
For more information, check out a related article by clicking here.
The forecasting and prediction features for passenger flow data, powered by machine learning, are integrated into the VISION MOBILITY reporting software. To learn more about this software and how it optimally utilizes data from counting systems, book an appointment with our expert via the link below.