In the field of rail transport, operational efficiency is essential for ensuring reliable and punctual service. One of the main concerns for operators is managing load peaks, which can lead to delays, congestion, and a decline in service quality. Thanks to advances in artificial intelligence (AI) and predictive analysis, it is now possible to anticipate and manage these load peaks more effectively.
Understanding predictive analysis with AI
Predictive analysis is a branch of AI that uses statistical techniques and machine learning algorithms to forecast future events based on historical and real-time data. In the context of railway vehicles, predictive analysis allows operators to accurately predict demand fluctuations and load on the network, enabling them to take preventive measures to avoid potential issues.
Data collection
The first crucial step in predictive analysis is collecting relevant data. Railway operators gather a variety of data, including train schedules, GPS location data, passenger information, weather conditions, and infrastructure status data. This data is then aggregated and analyzed to identify trends and patterns that may indicate imminent load peaks.
Data modeling
Once the data is collected, operators use data modeling techniques to create predictive models. These models are powered by machine learning algorithms that examine historical data to identify relationships and correlations between different variables. By adjusting and refining these models as new data becomes available, operators can continuously improve their predictive capabilities.
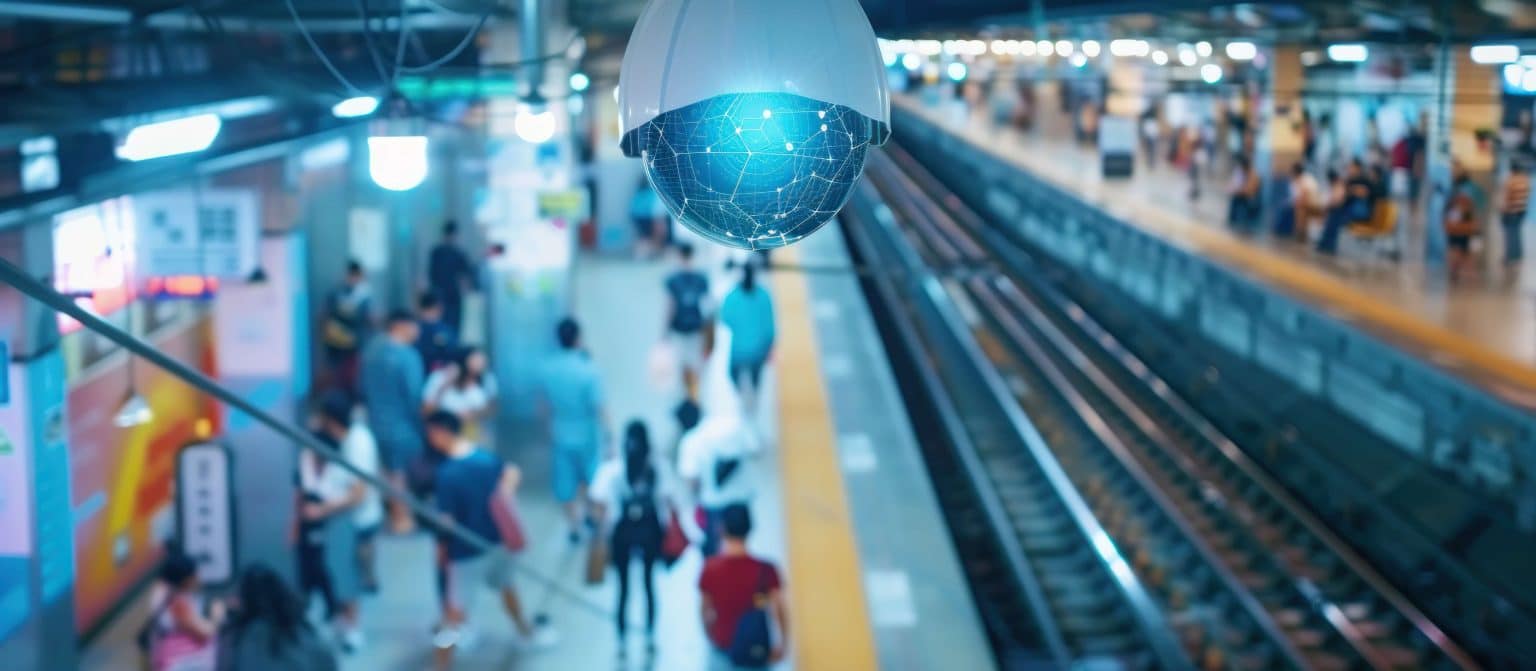
Forecasting load peaks
Once predictive models are in place, operators can start forecasting load peaks with impressive accuracy. By analyzing incoming real-time data and comparing it to predictive models, operators can identify emerging trends and take proactive measures to mitigate the effects of load peaks. This can include adjustments to train schedules, additional staff assignments, or changes in rail traffic management.
Advantages of predictive analysis
Using predictive analysis to anticipate load peaks offers numerous benefits for railway operators.
💰 Cost reduction
By anticipating load peaks, railway companies can better manage their resources, reduce operational costs, and avoid unnecessary expenses. For example, instead of permanently mobilizing additional trains, they do so only when necessary.
👍 Improved passenger experience
AI enables more efficient use of available resources, both human and material. Teams can be deployed more effectively, and trains can be used at full capacity without overloading the network.
⚙️Resource optimization
AI allows for more efficient use of available resources, both human and material. Teams can be deployed more effectively, and trains can be used at full capacity without overloading the network.
♻️ Reduced environmental impact
By optimizing schedules and capacities, AI contributes to more efficient energy use and reduced carbon emissions. Fewer empty or underutilized trains mean a smaller ecological footprint.
For more information, click here